Artificial intelligence (AI)
Artificial Intelligence has brought unprecedented opportunities and advancements but also challenges that require responsible and ethical deployment.
Ensuring transparency, addressing biases and fairness, protecting privacy and security, safeguarding against unintended consequences, and grappling with ethical implications are key challenges of AI.
Developing robust frameworks and guidelines prioritizing human well-being and mitigating risks associated with AI requires a collaborative effort among researchers, policymakers, and society.
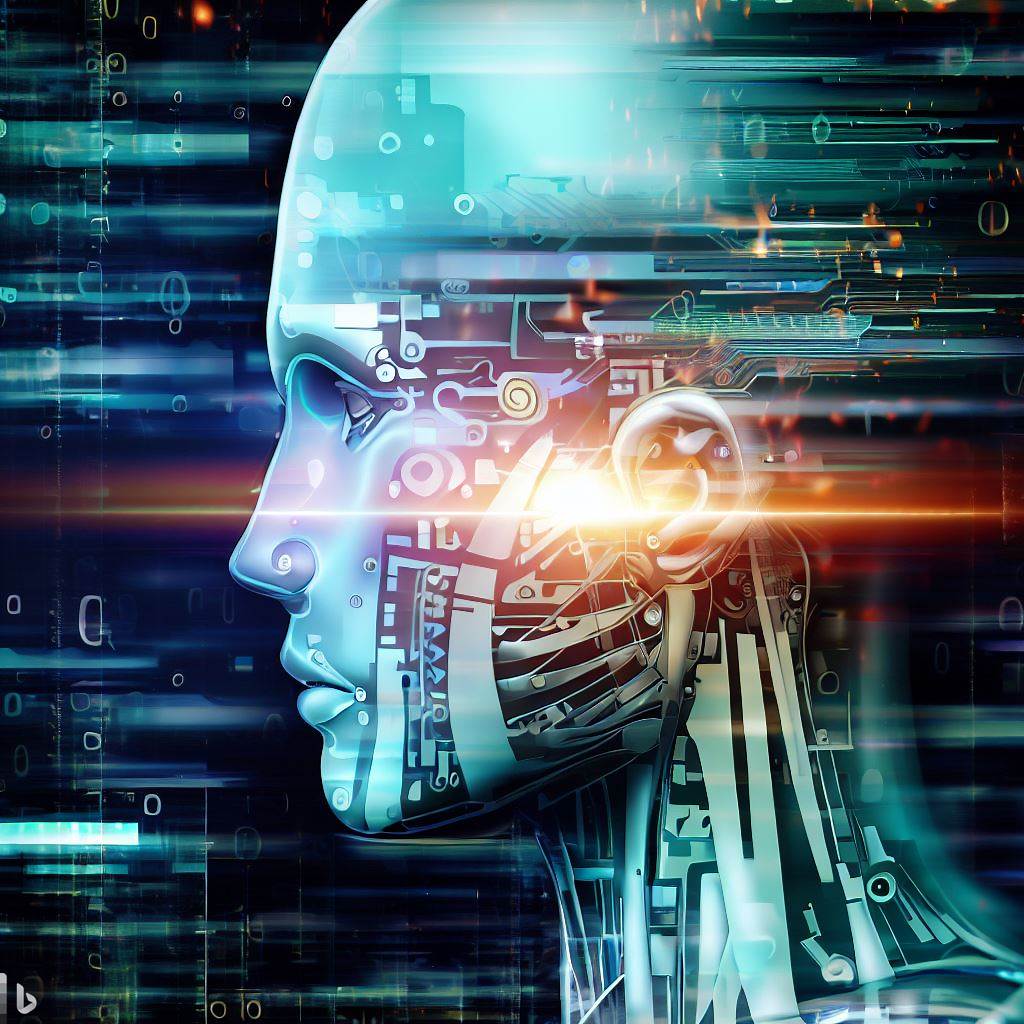
Artificial intelligence (AI) comes with certain risks and threats that need to be acknowledged and addressed.
Some of the risks include and are not limited to bias and discrimination, job displacement, privacy and security, Autonomous Weapons (development of autonomous weapons systems powered by AI ), lack of transparency.
AI-powered technology is capable of producing realistic fabricated content, like deepfakes and disinformation campaigns, that can result in widespread dissemination of false information and manipulation of public opinion.
It is imperative that AI systems be subjected to human oversight to prevent any catastrophic consequences.
Types of AI
There are generally four types of artificial intelligence (AI) based on their capabilities and level of human-like behaviour.
Narrow AI refers to AI systems designed to perform specific tasks with a narrow focus. These systems excel at specific tasks but lack the ability to generalise beyond their predefined scope. Examples of narrow AI include voice assistants like Siri and Alexa, image recognition systems, and recommendation algorithms.
General AI, also known as strong AI or artificial general intelligence (AGI), refers to AI systems that can understand, learn, and apply knowledge across various domains and tasks, similar to human intelligence. These systems could perform any intellectual task that a human can do. General AI is still largely theoretical and has not been fully realised.
An AI system with artificial superintelligence (ASI) outperforms human intelligence in almost every way. ASI would possess cognitive abilities far beyond human capacity and could potentially outperform humans in any intellectual endeavour. ASI is a hypothetical idea whose evolution and ramifications are the topic of intense discussion and conjecture.
Machine Learning (ML) is a branch of artificial intelligence that focuses on developing algorithms and models that can learn from data and make predictions or judgements based on it. Machine learning (ML) algorithms are trained on large datasets to look for patterns, generate accurate predictions, or perform specialised tasks. This covers methods such as reinforcement, unsupervised, and supervised learning. Numerous applications, including image identification, natural language processing, and recommendation systems, heavily rely on machine learning.
Some popular forms of AI
Hidden
Generative AI
Generative Artificial intelligence (AI) can create new content, such as images, writing, and music, based on trends and instances from previously collected data.
Pros of Generative AI:
- Creative Content Generation: Generative AI can produce original and creative content, including artwork, music, and even fictional stories.
- Data Augmentation: Generative AI can generate synthetic data and augment existing datasets for training machine learning models, improving performance.
- Enhanced Personalization: Generative AI can generate personalised recommendations tailored to individual preferences and interests, improving user experiences in areas such as e-commerce and entertainment.
- Exploration of New Possibilities: Generative AI can explore new design spaces and generate novel solutions, enabling innovative designs and ideas that may not have been considered.
Cons of Generative AI:
- Ethical Concerns: Generative AI raises ethical concerns regarding the authenticity and ownership of generated content. It can be misused for creating deepfakes, spreading misinformation, or infringing intellectual property rights.
- Biases and Unintended Outputs: Generative AI models can inadvertently perpetuate biases in the training data, leading to biased or discriminatory outputs.
- Data Dependency: Generative AI models require high-quality training data to produce satisfactory results. Limited or biased training data can impact the quality and diversity of generated content.
- Control and Interpretability: The black-box nature of many generative AI models makes it challenging to understand and control their decision-making process. This lack of interpretability can raise concerns in critical domains like healthcare or finance.
- Generative AI presents both exciting possibilities and challenges. Addressing the ethical considerations, biases, and control issues associated with its applications is crucial as it advances.
General AI
General AI, also known as Strong AI, refers to AI systems that possess human-level intelligence and can perform any intellectual task that a human can do.
Pros of General AI:
- Can handle a wide range of functions and adapt to new situations
- Potential for groundbreaking advancements in various fields
- Possibility of solving complex problems and making significant discoveries
Cons of General AI:
- Ethical and safety concerns as it may outperform humans in many domains
- Potential loss of jobs and economic disruptions due to automation
- The development of General AI is still a distant goal and poses significant technical challenges.
Machine Learning
Machine Learning (ML) is a subset of AI that focuses on enabling systems to learn and improve from experience without explicit programming.
Pros of Machine Learning:
- Ability to process large volumes of data and extract patterns and insights
- Effective in making predictions, classifications, and recommendations
- Can adapt and improve performance over time with more data and feedback
Cons of Machine Learning:
- Dependency on high-quality and diverse training data
- The black-box nature of complex ML models makes them difficult to interpret
- Potential for biased decisions if the training data contains biases
The advantages and disadvantages of various AI types are just a few examples. It is crucial to thoroughly analyse AI deployment’s ethical, societal, and technical consequences as it evolves.
Narrow AI
Narrow AI, also known as Weak AI, is designed to perform specific tasks and has a limited scope of functionality.
Pros of Narrow AI:
- Highly specialised and efficient in performing specific tasks
- Can automate repetitive and mundane tasks, improving productivity
- Can provide valuable insights and predictions in specific domains.
Cons of Narrow AI:
- Lacks general intelligence and cannot adapt to new tasks or situations
- Dependent on extensive training data and may not generalise well
- Prone to biases if not trained on diverse and representative datasets
Check out our AI and Machine Learning in Cyberecurity page to know the latest trend and predictions.